Author-Controlled AI™
Unlike Generative AI, which creates it's own answers based off millions of unknowable sources, Controlled-AI is trained on your content and can only deliver your validated answers. When accuracy, autonomy, and trust matter, industries choose Controlled-AI.
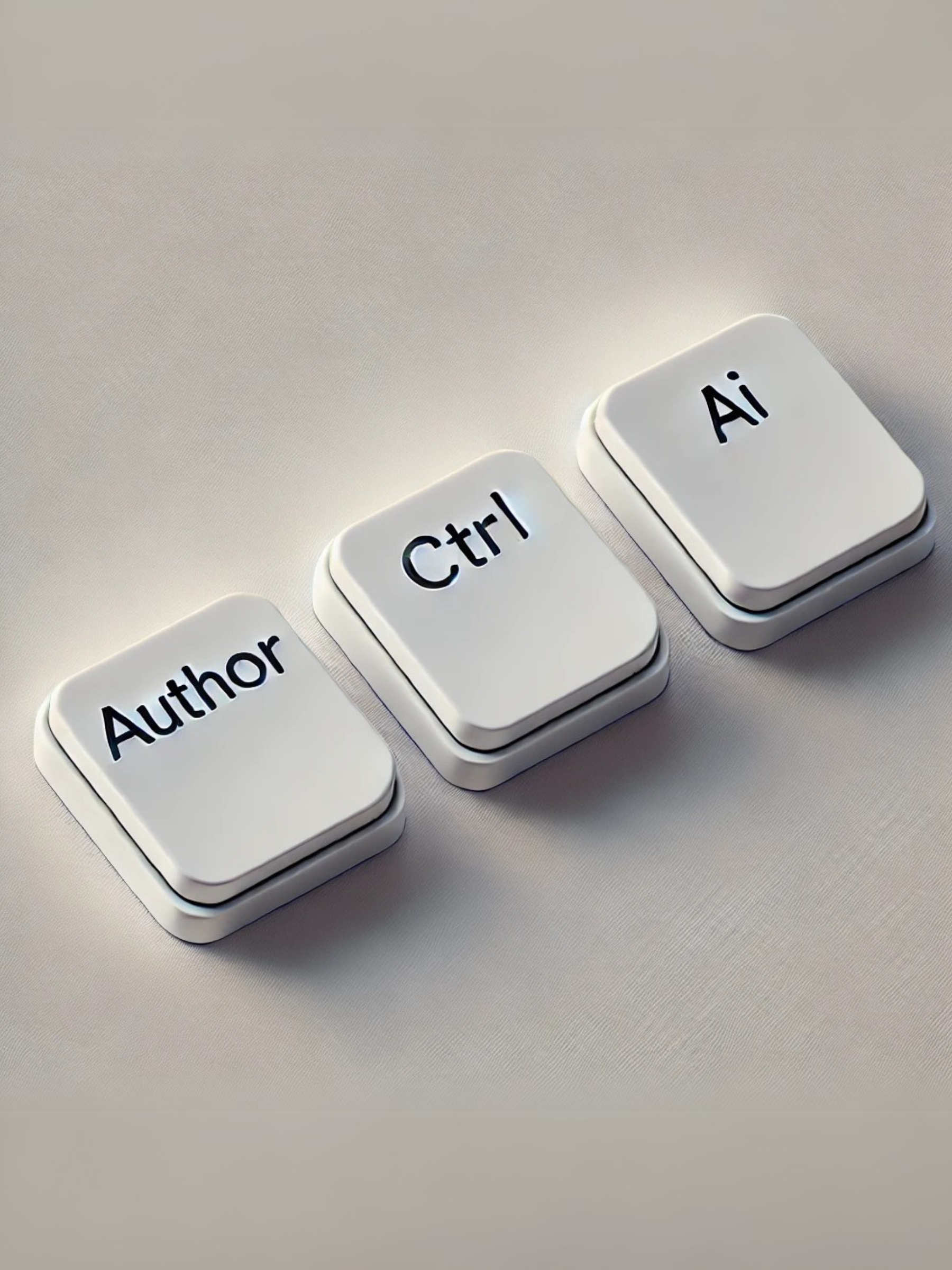
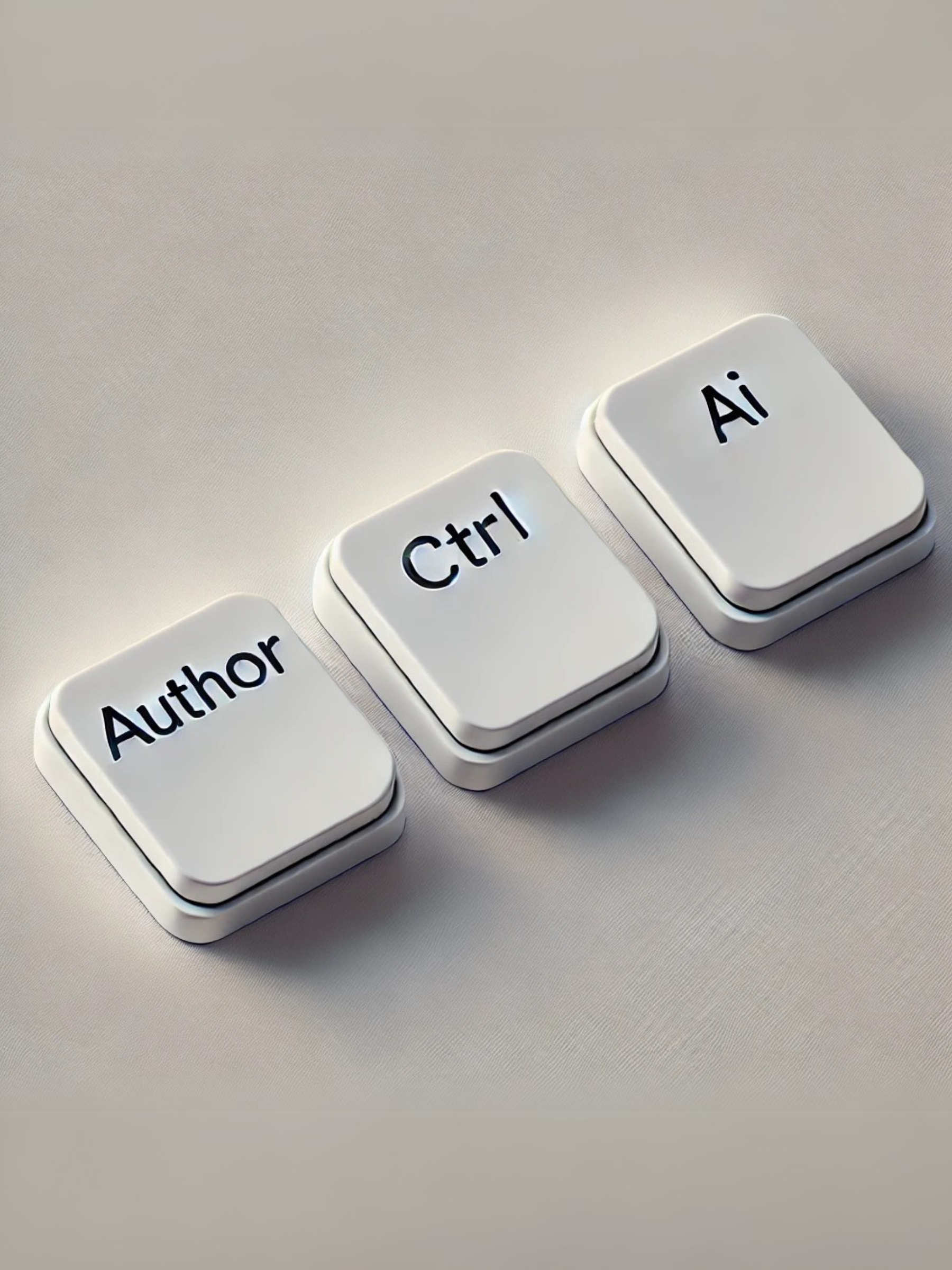
The difference between controlled and generative AI - it's all about risk.
When your message matters, you need to remain in control. Let's begin by understanding the core difference between generative and controlled AI and which is better suited for what type of tasks.
What is Generative AI or GENAI?
Generative AI refers to a category of artificial intelligence techniques and models designed to generate new data that resembles or mimics existing data. Unlike traditional AI systems primarily used for classification or prediction tasks, generative AI focuses on creating novel outputs, such as images, text, audio, or video, based on patterns learned from training data. Generative AI encompasses various approaches, including:
1. Generative Adversarial Networks (GANs): GANs consist of two neural networks, a generator and a discriminator, trained simultaneously in a competitive manner. The generator creates synthetic data samples, while the discriminator distinguishes between real and fake data. GANs can generate highly realistic and diverse outputs through this adversarial training process.
2. Variational Autoencoders (VAEs): VAEs are probabilistic generative models that learn to encode and decode data samples. They consist of an encoder network that maps input data to a latent space and a decoder network that reconstructs data samples from latent space representations. VAEs can generate new data samples by sampling from the learned latent space.
3. Autoregressive Models: Autoregressive models, such as recurrent neural networks (RNNs) or transformer architectures like GPT, generate sequences of data one element at a time, conditioned on previous elements. These models are commonly used for generating text, music, or other sequential data.
Generative AI has applications in various domains, including image generation, text generation, content creation, data augmentation, and more. Overall, generative AI enables the creation of novel and diverse data samples, pushing the boundaries of creativity, imagination, and innovation in artificial intelligence.
What are the top risks of GENAI today?
Bias / Misinformation: Generative AI, like any technology, can reflect biases present in the data it's trained on or the algorithms used to create it. The AI model may produce biased or skewed outputs if the training data is biased or incomplete. Bias can manifest in various ways, such as reinforcing stereotypes, favoring specific demographics, or misrepresenting underrepresented groups.
Hallucinations
"Generative AI hallucinations" are often used to describe unexpected or surreal outputs generated by AI models, particularly in the context of generative models like Generative Adversarial Networks (GANs) or autoregressive models like GPT. These "hallucinations" occur when the AI model generates unusual, imaginative, or divergent outputs from what it was explicitly trained on. They can range from distorted images to nonsensical text passages to new concepts that emerge from the model's creative processes. Hallucinations can sometimes be intriguing and lead to novel insights, but they can also be nonsensical or even disturbing, depending on the context and the quality of the model. In the case of text-based generative AI like GPT, hallucinations might appear as sentences or paragraphs that drift away from coherent discourse, producing unexpected associations or blending concepts in unusual ways.
Ethical considerations
There are several ethical considerations with the use of GenAI, including bias, fairness, manipulation, privacy concerns, threats to intellectual property rights, transparency and the concept of human empowerment versus replacement.
While generative AI has the potential to enhance human creativity and productivity, there are concerns about its impact on employment and the economy. Balancing the benefits of automation with the need to ensure equitable distribution of opportunities and resources is a significant ethical consideration.
Addressing these ethical considerations requires collaboration among stakeholders, including AI researchers, policymakers, ethicists, and industry leaders, to develop guidelines, regulations, and best practices that promote the responsible development and deployment of generative AI technologies.
What is Controlled AI?
Controlled AI is an artificial intelligence system designed or programmed to operate within predefined constraints or guidelines. These constraints can include limitations on the actions the AI system can take, the types of decisions it can make, or the range of inputs it can process.
Controlled AI aims to ensure that AI systems behave predictably and responsibly, particularly in sensitive or high-stakes applications with significant potential for unintended consequences or misuse. By imposing constraints on AI behavior, developers can mitigate risks related to safety, security, fairness, and ethical concerns. Examples of controlled AI include:
Safety-Critical Systems
AI systems deployed in safety-critical domains, such as autonomous vehicles, medical devices, or industrial control systems, often operate under strict constraints to ensure the safety of users and the general public.
Content Moderation
AI algorithms used for content moderation on social media platforms are often designed to enforce community guidelines and prevent disseminating harmful or inappropriate content.
Compliance and Regulations
AI systems operating in regulated industries like finance or healthcare must comply with industry-specific regulations and standards. Controlled AI ensures that these systems adhere to legal and ethical requirements.
Human-in-the-Loop Systems
Some AI systems are designed to work in conjunction with human operators, who provide oversight and intervene when necessary to correct errors or ensure compliance with organizational policies.
Bias Mitigation
Controlled AI techniques can mitigate biases in AI models by constraining their behavior to avoid producing discriminatory outputs or reinforcing existing biases in the training data.
Overall, controlled AI approaches are essential for building trust in AI systems and ensuring responsible deployment across various domains. By defining clear boundaries and constraints, developers can mitigate risks and maximize the benefits of AI technology while minimizing potential negative impacts.
Qvio® allows you to upscale your video content by adding Author-Controlled AI™ to handle viewer questions in real-time. However, the human-in-the-loop approach requires the author to authenticate generated answers in the data set before publication.
Why we use both
While Qvio leverages controlled AI for answer delivery, authors can jump-start their answer data with GenAI or connect to another trusted LLM. However, before the controlled AI model can consider those answers, the author must validate them. This approach allows content creation that's quick and easy to scale and content delivery that is trustworthy and safe.
- Improve model accuracy in response to gaps
- Build trust with valuable insights into how your models are performing
- Continually improve the fairness of model outcomes without re-deploying
- Comply with industry regulations governing use of AI
Upload your video, generate your dataset, refine and share!
Ready to learn more?